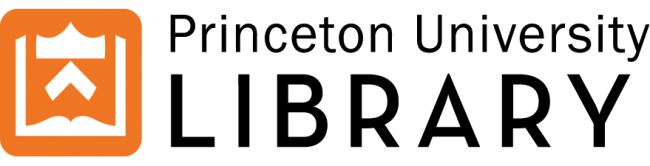
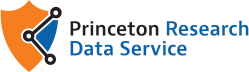

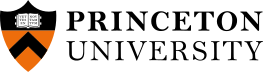
The multi-scale, mutli-physics nature of fusion plasmas makes predicting plasma events challenging. Recent advances in deep convolutional neural network architectures (CNN) utilizing dilated convolutions enable accurate predictions on sequences which have long-range, multi-scale characteristics, such as the time-series generated by diagnostic instruments observing fusion plasmas. Here we apply this neural network architecture to the popular problem of disruption prediction in fusion tokamaks, utilizing raw data from a single diagnostic, the Electron Cyclotron Emission imaging (ECEi) diagnostic from the DIII-D tokamak. ECEi measures a fundamental plasma quantity (electron temperature) with high temporal resolution over the entire plasma discharge, making it sensitive to a number of potential pre-disruptions markers with different temporal and spatial scales. Promising, initial disruption prediction results are obtained training a deep CNN with large receptive field ({$\sim$}30k), achieving an $F_1$-score of {$\sim$}91\% on individual time-slices using only the ECEi data.
Show MoreFilename | Size |
---|