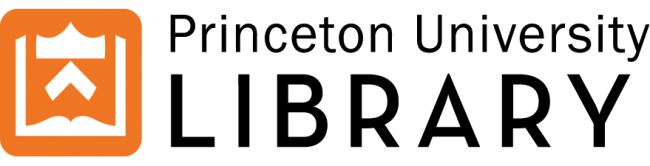
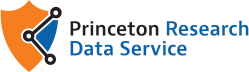

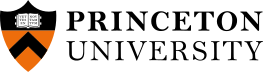
This dataset contains input and output files to reproduce the results of the manuscript "Homogeneous ice nucleation in an ab initio machine learning model" by Pablo M. Piaggi, Jack Weis, Athanassios Z. Panagiotopoulos, Pablo G. Debenedetti, and Roberto Car (arXiv preprint https://arxiv.org/abs/2203.01376). In this work, we studied the homogeneous nucleation of ice from supercooled liquid water using a machine learning model trained on ab initio energies and forces. Since nucleation takes place over times much longer than the simulation times that can be afforded using molecular dynamics simulations, we make use of the seeding technique that is based on simulating an ice cluster embedded in liquid water. The key quantity provided by the seeding technique is the size of the critical cluster (i.e., a size such that the cluster has equal probabilities of growing or shrinking at the given supersaturation). Using data from the seeding simulations and the equations of classical nucleation theory we compute nucleation rates that can be compared with experiments.
Show MoreThis dataset is too large to download directly from this item page. You can access and download the data via Globus
Show MoreFilename | Size |
---|